It used to be that when someone said “AI”, images of sci-fi films came to mind.
Nowadays, the effect is more prosaic: it’s become de rigeur to use AI as shorthand for “business wonder cure”. When we hear “AI”, we know we’re supposed to see it as the answer to any and all business problems, whatever the industry.
At the same time, we’ve seen Customer Experience become the key differentiator in all sorts of sectors, but particularly retail. We all want to be treated as individuals and expect those we buy from to act as value-add service providers, not just vendors. That fact has fuelled the rise of AI as the perceived silver bullet for retailers – the more data you have at your disposal and the faster you can put it through effective analysis, the more likely it is that you’ll provide a beguiling, personalised Customer Experience and product offering.
However, AI is a term that’s used too freely. It has become confused with machine learning, losing its original meaning of a completely independent decision-making engine. True AI would make decisions based on so much information that you couldn’t know the decision it’s going to make before it makes it – much like a human being.
In contrast to that, current machine learning applications are very controlled – they put a set data flow through a known algorithm which auto-updates based on the results it receives, but they’re nowhere near the level of independence required for true AI.
Eliminating bias
There are good business reasons why AI remains in the realm of hype rather than frontline use, though. Financial services firms aren’t using AI, for example, because they can’t control its results – there’s too high a risk that mortgage applications could be affected by unconscious bias, for example.
The same risks apply to retailers. A good example of this played out a few years back when Amazon attempted to take the human element out of hiring. It took data about the type of people it had previously hired, processed it, and then set the resulting algorithm to hiring people. However, it ended up only hiring men – because that was what Amazon had historically done. It discounted women based on past experience, and therein lies the problem. AI based on past data doesn’t let you change for the better.
This kind of coded bias is also indicative of the people who’ve made the algorithm – if it’s a non-diverse group designing the algorithm, they’re more likely to almost accidentally code bias in. This is one of the main reasons why pure AI isn’t often used – the data you have might be biased, and you won’t know until it’s running. If it goes wrong, you can’t unpick it.
Think of it like a child growing up: once they’ve picked up negative behavioural traits, it’s very difficult to undo the hardwiring.
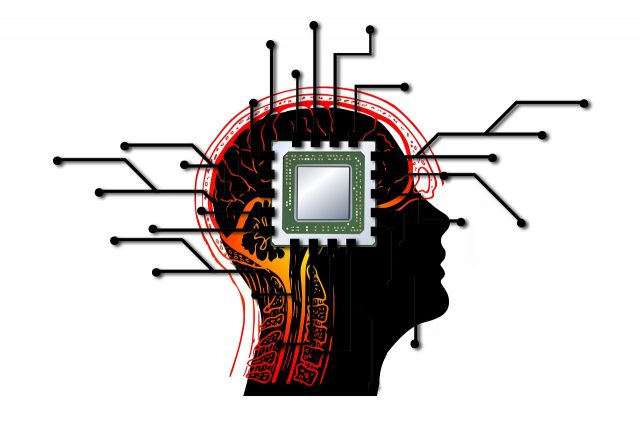
Obtaining data
Despite all this, genuine AI is a possibility. The key is ensuring that applications have access to sufficient data – and the right kind of data. The more data you have, the more likely it is that you can build something that will provide accurate, non-biased results.
Unfortunately, we’re simultaneously seeing a sharp decrease in willingness to share data, as scandals like the Cambridge Analytica debacle damage confidence in the safety and privacy of large-scale data sharing. As a result, businesses have to navigate a very fine line between feeding enough data into their automated systems to achieve an optimal, non-biased Customer Experience and preserving customers’ privacy and trust.
Take the worked example of calling HMRC. As most of us will know, it can often take forever – it’s not a positive experience. To solve that problem, HMRC recently announced a desire to use voice biometrics for identification to speed up the process and remove the endless questioning. The system would be able to tell your mood, quickly identify why you’re calling, and put you through to exactly the right person to solve your problem.
The idea is a good one, but it depends entirely on people being comfortable sharing their voice data. Without that data, how do you optimise the system? As we move away from data-sharing as the default, how can companies still optimise Customer Experience with AI-driven technology?
Prioritising end users
In a nutshell, the answer is preparation. Lots of organisations come to my practice and say they want their customer journey to take advantage of AI and machine learning, but often they don’t have the tools in place to support it – no security, no data automation, and no clear understanding of the business need they’re trying to address.
They’re starting from the wrong point. Technology is not the end goal – Customer Experience is.
Companies need to start their AI CX journey with basic identity and access management. They need to inform their customers that they value their data and their security, and that they’re going to use their data with set controls in order to then keep them informed and give them proactive customer service, say.
Transparency is key – and you have to have the technology in place to back it up.
The bottom line is that an AI-enabled customer journey should always start with security. It’s essential to be able to protect your customer’s data as you use it. Not only that, but you need to make sure you’re providing a service that actually meets real needs, so find out what your customers want.
Start with their needs, not your technological goals. AI can take customer relationships to the next level, but it must be designed with the end user in mind.