The application of the computational analysis and learning techniques described in previous research, manifest themselves in the form of artificial intelligence (AI). AI represents the ambition to create machines that can think, learn and create solutions to problems with the same range to which the human mind can be applied.
AI is absolutely nothing new – all of us are using it every day. Every time we send an email, use a credit card or travel or search the Internet, AI systems are the bedrock on which we perform these activities. Intelligent algorithms are constantly checking and detecting credit-card fraud, flying and landing aeroplanes, keeping track of inventories and even manufacturing products in robotic factories.
Genetic algorithm
These AI algorithms are based on machine learning, deep learning, artificial neural networks and natural language processing. Another component of AI is the algorithms that are used to make them. A good example of this is a genetic algorithm (GA), which is a heuristic search method used in artificial intelligence and computing based on Darwin’s theory of evolution. GA is used for finding optimised solutions to search problems based on the theories of natural selection and evolutionary biology, i.e., selection, mutation, inheritance and recombination.
GA is based on the classic view of a chromosome as a string of genes. R.A. Fisher used this view to found mathematical genetics, providing mathematical formula specifying the rate at which particular genes would spread through a population.
When solving constrained and unconstrained optimisation problems, a classical algorithm generates a single data point at each iteration. The sequence of data points then approaches an optimal solution. By contrast, GA uses a process similar to natural selection – it repeatedly modifies a population of individual solutions and at each step, randomly selects data points from the current population of points and uses them as “parents” to produce the children for the next generation. Over successive generations, the population “evolves” toward an optimal solution.
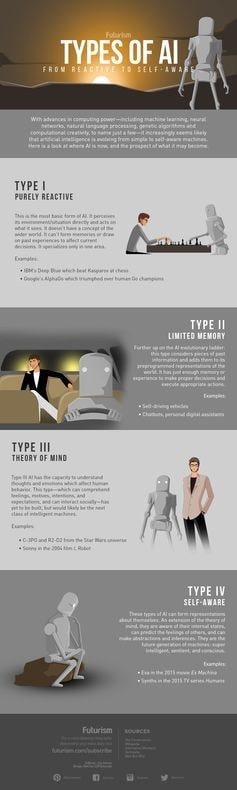
Although randomised, GAs are by no means random. Instead, they exploit historical information to direct the search into the region of better performance within the search space. GA effectively simulates the survival of the fittest among individuals over consecutive generations for solving a problem. Every one represents a point in a search space and a possible solution. The individuals in the population are then made to go through a process of evolution.
It is via the juxtaposition of GA, machine learning, deep learning, artificial neural networks and natural language processing that AI can learn from data and create solutions – a fundamentally human activity. Today, these methods are used for searching through large and complex data sets to find reasonable solutions to complex issues by solving unconstrained and constrained optimisation issues. They are used to solve problems that are not well suited for standard statistical models including problems in which the objective function is discontinuous, non-differentiable or highly nonlinear. They can also be used on mixed (continuous and discrete) combinatorial problems, as they are less susceptible to getting ‘stuck’ at local optima than classical gradient search methods.
Narrow and general AI
These advances have allowed us to leverage the gargantuan amounts of data being produced in any format to perform a plethora of actions. However, most of the AI performing these tasks are “Narrow AI” (ANI), as in they are devised and constructed to perform a very specific function. They are capable of performing isolated tasks that are computationally complex but well-defined. Examples such as these include IBM’s Watson, self-driving cars and chatbots.
These AI’s, although high-performance, are hard to generalise to new problems. They are tools that have been made for a very specific goal (e.g., win a chess match and answer customer queries). A general artificial intelligence (AGI). however, is constructed to have a more general goal – learn new things, self-improve, expand the scope of functionality, or even create something new. AGI systems are explicitly designed to autonomously learn novel tasks and adapt to changing environments. They have been developed to have open ended goals. Even the programming language for AGI’s are constructed under this philosophy. Replicode is one example of an AGI language – unlike other AI languages, it is designed in the form of short parallel programs that no explicit conditional statements like “if-then” statements or loops.
Business implications
AI and AGI are being used in a host of sectors. Last year, Uber acquired the AGI startup Geometric Intelligence and intends to create a new artificial intelligence research arm at its headquarters. What Uber intends to do with the purchase is anyone’s guess and dissecting the way business plans will change with these technologies is an ongoing process. But one thing is clear, the work landscape is in a state of turbulent flux and adapting to these changes is the only way to ensure economic survival. As stated by Uber’s chief product officer Jeff Holden:
“If you look into the future, there are going to be step-function changes in artificial intelligence that will affect business models and business opportunities.”
But progress is being made and financial firms, which are already in the process of creating hedge funds that can predict price changes based on a host of data, including prices and volumes, news and social media data in various languages and other economic and accounting data at national and company levels. Tents of economic theory such as elasticity, market structure, price optimisation or even aggregates, in general, are all exposed to a profound transformation in the eyes of AI.
We are about to witness much wider implications of AI on the economy, society and business. The learning journey has just begun for humanity to confront itself with the pace of peaceful pervasion of AI and the rise of new horizons that the integration of this technology will vehemently shape its image and resemblance.
Written by:
Mark Esposito, Fellow, Judge Business School, University of Cambridge
Kariappa Bheemaiah, Associate research scientist, Cambridge Judge Business School
Terence Tse, Associate Professor of Finance, London campus of ESCP Europe Business School
Source: We Forum